According to the European commission, half of the energy consumed in the European Union goes to heating and cooling of buildings [1]. According to Conolly et al. [2], the current market share of district heating (DH) in EU's building stock is only 13 %. In addition, Werner [3] states that the expansion of DH is possible and would decrease carbon dioxide emissions in the heating systems through improved energy efficiency. Also, Persson and Werner [4] noted that there is a possibility to increase this market share to cover more buildings and this could be done economically in many occasions.
Because the energy consumption of DH systems is so great, there is a high priority to make them more efficient. In the framework strategy for a resilient energy union, the commission states that there are huge efficiency gains to be captured in the district heating sector [5]. There is also strong support from the commission for the smart cities and communities' initiative that focuses on creating smart cities using telecommunications and digital networks to improve efficiency [6]. District heating and cooling networks must evolve with other systems to create smart cities. These smart energy systems are described by Lund et al. as a system where electricity, thermal and gas grids are managed together to achieve optimal solutions for each separate grid and for the whole system. Furthermore, Lund et al. state that the ability to incorporate several different sources of heat, such as waste heat and heat pumps, and increased efficiency of DH systems play an important role in the heating systems of tomorrow. The reduction in supply temperature is a key factor in decreasing network heat losses thus improving the overall efficiency of the heating. This also promotes the use of waste heat. [7].
In Finland the construction of DH systems started after the 1940's [8]. DH has evolved into the most common heating form of buildings especially in cities with a total market share of 46 % [9]. However, the systems are still using quite high temperatures and would be categorized as 3rd generation DH networks according to Lund et al. The temperatures in the 3rd generation networks are often below 100 °C but can be as high as 120 °C in the winter season.
Lately DH networks have also been seen as platform for demand side management (DSM) and the utilization of waste heat [7]. DSM is a technology where energy consumption of a building is altered without noticeable effect to the inhabitant. This is done by taking into account buildings' thermal attributes and the weather forecast. For example, the peak hours of heat consumption can be shifted or the consumption profile flattened by taking advantage of the heat stored into the building mass.
In the future, there will be a need for smart heating networks and they could even play a role in balancing the electricity networks as more intermittent renewable electricity production is increased [10]. Smart heating systems will connect the production and consumption sides into a system that is more efficient compared to the traditional one. In a smart heating network, the customers collaborate with the energy utility and even become prosumers who produce energy to the network. These developments require new business models and novel control methods for the system to benefit all parties. In addition, new technologies also have to be implemented in buildings, such as more efficient heat exchangers and online measurements. The modelling of areas more accurately will also be one requirement for smart heating systems. On the other hand the smart heating system itself will produce more data for analysis than a conventional heating system.
Studies have been done on cost formation for local production from spatial cost point of view [11]. In the study a link between the location of the local production in relation to the consumption was found. In addition, marginal cost formation of district heating has also been studied in [12] where significant savings for the production system were found when incorporating external heat producers. However, this study noted that heat distribution issues in networks and the spatial nature of production units was not taken into account. In [13] marginal pricing of heat was studied and results indicated that total production costs and emissions decreased when waste heat was incorporated into the system. The study also noted that heat pump production might benefit from lower district heating temperatures.
Prosumers in DH networks have also been studied in [14] and their potential was considered significant. Although, this study noted the need of a closer environmental research into a specific area. Potential for excess heat delivery to DH networks have been studied in Sweden [15] and Japan [16] where significant potential was found. In addition, excess heat potential mapping has been also done on a country level [17] and on a regional level [18]. In Denmark sources of waste heat were found to be present near almost all studied DH networks and that they could play a significant role in the heating systems of the future [18]. However, the study mapped the proximity of the waste heat source in relation to the areas with DH networks but did not take DH network specific attributes into consideration.
The sources of distributed production of excess heat to DH networks are usually coming from different types of buildings, such as industrial and domestic buildings, shopping centers, office buildings, hotels, indoor ice skating rinks and supermarkets. Utilizing this kind of local excess heat has been seen as beneficial since it decreases costs and reduces the use of combustible fuels and emissions [16, 19, 20,]. In addition to waste heat utilization, DSM has been studied on a building level [21] as well as system level [22] in order to understand the potential and the effects of the actions. However, these studies do not fully take into account the spatial nature of the DH system and the challenges it brings. This issue was also noted by Syri et al. in [12] by stating that in a network that covers a large geographical area distribution issues have to be considered. Also Kontu et al. noted the issues arising from the spatial nature of the DH network in [22].
These previously mentioned studies have all noted the benefits of either excess heat production or customer side activation in the form of demand side management or prosumerism, but there is a need for closer analysis of the heat networks themselves in order to understand what drivers are there for maximizing local production.
Local heat production is usually made with heat pumps from some kind of waste heat source, for example waste heat from cooling. The challenges regarding heat pump production into DH networks are usually economical. The local production has to be primed into higher temperatures so it can be utilized in the network and then it needs to be delivered to the location where energy is needed. This causes expenses in form of investments and operational costs. Mismatch between the waste heat temperature and the DH network temperature, which hinders utilization has also been recognized in literature [7].
Sometimes local heat pump production is not profitable because DH companies are buying the excess heat at a price level compared to their own production costs that can be quite low. For example, combined heat and power (CHP) production with biofuels is subsidized in Finland and this leads to low heat production costs. DH companies then use only this price as a reference price for excess heat without taking into account the positive effect on the DH network.
Sometimes the price is so low that real estate owners do not have incentive to invest in excess heat production, especially, if the required temperature levels are high compared to the economical production of the heat pump. However, the proliferation of heat pump technology utilization is driven by technological advances, increasing prices of energy and legislation regarding energy efficiency and environmental protection [23]. If the local production is static and heat is produced consistently around the year, the seasonality of DH in northern countries might be an issue; usually in the summer the heat demand is very low compared to the winter.
In [24] Brand et al. studied the effect of prosumers in a DH network concluding that when the locally produced heat is lower than the network temperature the local production can harmfully affect the neighboring customers.
Further research is required to better understand how the network topology in DH networks could be analyzed so that areas most suitable for distributed local production are recognized. If the reason for unexploited local production is indeed economic based on the current pricing models, price levels, DH temperature levels or the unprofitable investment into a larger heat pump for excess production a deeper understanding into the factors affecting such systems is needed.
Main research questions of this study are related to whether areas suitable for lower supply temperatures can be identified using traditional network simulation tools and what drivers make local heat pump production profitable. In addition, network heat loss and emissions impact of these actions is studied.
Favorable locations for local distributed heat production and attributes that promote local production are investigated by simulating an existing DH network in the 4th largest city in Finland, Vantaa. The favorable locations are those where the reduction in supply temperature is the largest in all studied scenarios. In addition, actual technical building data is analyzed with real customer substation data to determine suitable areas for production and temperature levels in the network that would maximize local heat production.
Customers' motivation to participate in local heat production through heat pumps are analyzed through net present value analysis where the heat pump investment needed for local production is optimized using hourly prices for electricity, heat purchase, surplus heat sales, capital and operational costs and network supply temperatures. The profitability is compared to the underlying real estate investment return targets determined by real estate market information.
It was found that by using the existing simulation tools available for the DH company favorable locations for local production can be found. In addition, by analyzing open source building data it is possible to determine areas which have suitable building stock for local production. It was also found that lower supply temperatures not only reduce emissions but also promote profitable local heat pump production significantly when the net present value of heat pumps in buildings is optimized. Local production was found to increase 1214 MWh which is 4,6 % of the total annual heat consumption of the area.
The methods in this article follow two steps. First, simulation of the DH network reveals areas with potential for low temperature networks. Static simulation of the DH network is conducted at key moments when production unit changes happen. Second, the profitability of investments into local heat production in the identified areas is determined by optimising the net present value (NPV) of local heat pump production.
Heat demand is seasonally oriented in heating networks, affecting the production of DH. DH systems usually have several production units, which are started based on their costs. This allows to divide the year into different time sets based on the production. The production data can be gathered into a duration curve, where distinct periods of production can be seen. The change in production scenarios affect the pumping areas since there is more energy to transfer and it is strongly affected by change in outside temperatures.
Usually DH systems have different pumping areas that serve the heat demand of buildings in that area. Whenever the heat demand changes, the production has to accommodate to this new demand by increasing or decreasing heat production and pumping power. This changes the balance of heat supply in the network.
To determine favorable locations for local production the network transfer of heat was simulated in different outside temperatures with a geographic information systems (GIS) model where every pipe, pumping station, production facility and customer DH substation have a geographical location. Also, the pipe characteristics are built into the model. This means that pipe size and length are also taken into consideration. The simulations were done with Trimble NIS. Trimble takes into account the DH pipe heat conductivity along with heat demand and outside temperature. For the calculations the Trimble program uses Neplan's heating basic module as a computing engine. [25]
The DH water temperature from the production facilities is based on the needs of the customers. Each customers DH substation has to have a certain DH water temperature in the corresponding outside temperature. The customer's geographical location set the need for certain temperature and flow from the production units. In this sense the customers on the edges of the network are critical in balancing the heat production.
The maximum potential for real estate owners to produce heat into the network was assessed with a calculator that optimizes the maximum heat pump installation (peak power) according to the profitability of the investment compared to real estate investor's return target. The size of the heat pump was calculated for each property type (retail, residential, logistics and office) and the heat consumption for each property type was the aggregated consumption of all buildings in the observed area. The consumption profiles were derived from real consumption data in the area weighting also for the building construction years.
The Heat pump sizing optimization follows the method presented by Kontu et al. [26]. In the method the current heat demand of a property (measured hourly over a year) is met with alternative heating solution (heat pump + electric boiler), and the size of the alternative solution is optimized so that the NPV of the investment is maximized. The annual net savings are calculated by deducting the costs of running the alternative solution from avoided DH costs. The paper presented the following formula (1) for the optimization.
(1)
Where HP, EB, EC are respective capital expenses for heat pump, electric boiler and larger electricity connection. The net savings from using heat pump is calculated with the following parameters: Hdh = consumed DH energy, Pdht = price (€/MWh) of DH at time t, Hhp = electricity required for HP, Heb = electricity required for EB, Pet= price of electricity, Hopen = energy sold to Open DH, Popent = price received for energy sold to Open DH, Ldht = load demand cost for DH, OPEX = operating expenses of HP, Et = fixed annual electricity capacity costs and r = used discount rate. A more detailed description of the method can be found in [26]. Since in this paper the heat that can be produced into the DH networks is analyzed more closely, the above method was altered so that the coefficient of performance (COP) changes when heat is produced into the network (in a higher temperature than needed for own consumption). Furthermore, basis scenario was calculated with one heat pump where if energy is produced into the DH network, the whole energy production for that hour is calculated with the lower COP (based on the DH supply water temperature). In the second scenario a second heat pump is installed (that increases the total capital expenses of the heat pump system by 25 %) that allows producing the first 50 °C of water with the higher COP and the rest with the lower COP (based on the supply temperature).
The DH network examination consist of real measurement data from a district heating network in Vantaa, Finland. The data was collected from a time span of one year from 2018. The data is actual hourly production data from each DH production unit, building related data, and customer substation measurement data. In addition to using this data the network was also modelled for simulations of heat losses in different production scenarios. Open source building data was used to assess the local heat production potential of buildings in selected areas. This data contains all buildings in the city and has information on the building properties such as purpose of use, year of construction, floor space and heating system.
Along with the building data, the actual heat consumption was also analysed to determine lower temperature levels for the selected area. District heating measurement data was collected from substations. This data includes hourly peak power, supply and return temperatures and water flow. All of the measurements are from the DH network side. No data was available from the building heating system side.
Input data for the local production optimization tool is described in more detail in Table A3 (Appendix A).
The DH system consists of several production units. There are two combined heat and power plants that have different production units. CHP 1 has one biofuel boiler and one coal boiler, each connected to their own steam turbine. There is also a gas turbine that is connected to a heat-recovery steam generator. CHP 2 has two waste-to-energy boilers that are connected to one steam turbine. CHP 2 also has a gas turbine that is connected to a heat-recovery steam generator. Both CHP plants also have heat storages that are used for balancing short term demand fluctuations. Heat only boilers (HOBs) are used whenever the heat demand is high or when a CHP plant is offline. The HOBs have gas as their main fuel and oil as a reserve fuel apart from HOB 2 that only uses oil. Peak power produced in 2018 was 654 MW and annual production was 1900 GWh. Length of the DH network was 564 km in 2018 [9]. More detailed information about the production units and the DH network is displayed in Table A1 (Appendix A).
An overview of the district heating system and the location of the production units can be seen in Figure 1. The locations of the CHP power plants are marked with red squares (CHP 1 and 2), pumping stations in blue squares (P 1-7) and HOB's in green squares (HOB 1-5). The black lines mark the different pumping zones in the network.
Overview of the DH network and production units
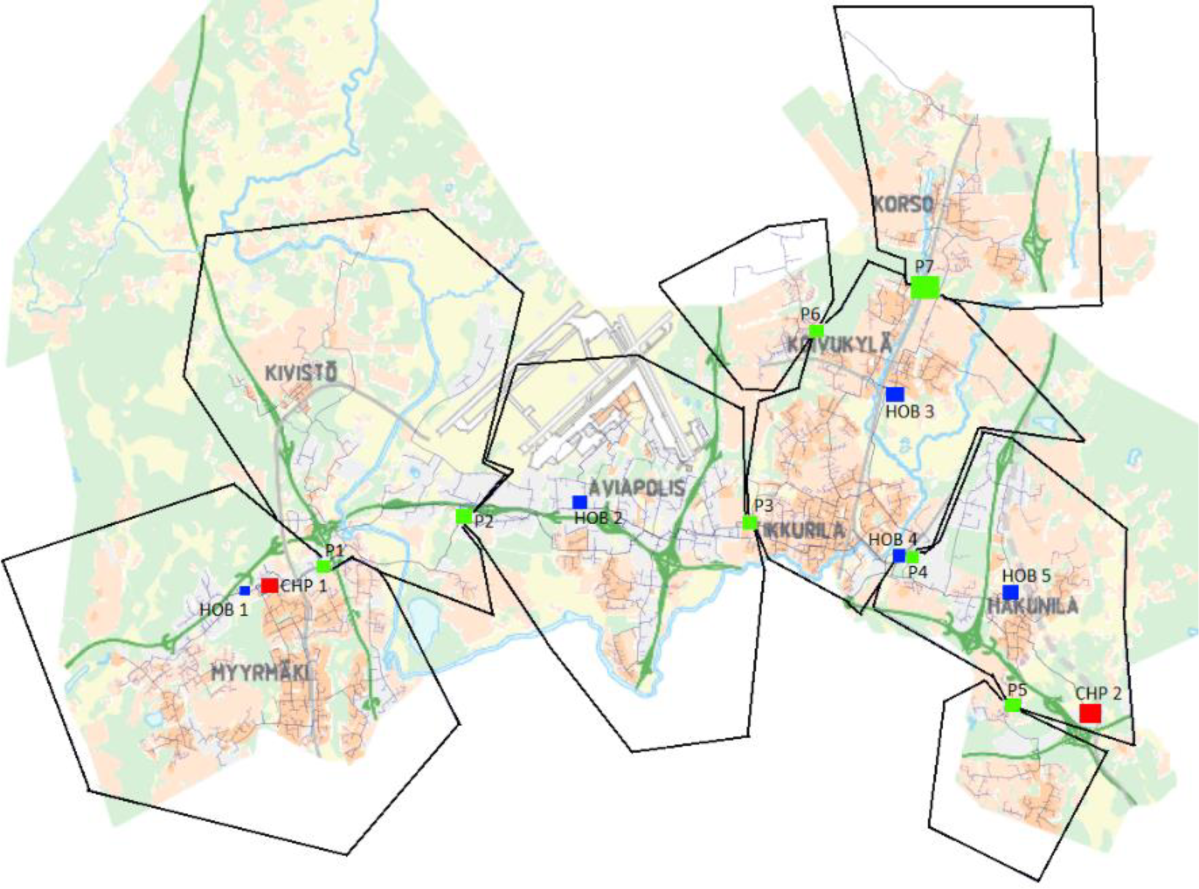
At every intersection of two pumping areas is a pumping station that has interchangeable pumping direction depending on the consumption and production of energy. The network is not built as a loop so the pumping areas do not change as the production is increased or decreased. However, some pumping stations are switched off when the production is decreased significantly in the summer and less pumping power is needed.
This section presents the identified locations favourable for local heat production, building specific data analysis, building heating system analysis, annual heat losses, potential for local production and the heat loss reduction resulting from lower supply temperatures and the following reductions in emissions.
Simulation periods were determined according to the before mentioned method of analysing the changes in production over a year. During the year, three distinct periods of production were found. In one, during the coldest period of the year, all different production facilities are in use. In the second, only two main CHP plants are in use and in the warmest period of the year mainly one CHP plant is used. These three scenarios affect the pumping areas since there is more energy to transfer. The change in production occurs at outside temperatures of -26 °C, +0 °C and +14 °C. These are the three periods at which the DH network is examined more closely in the static network simulation. The duration curve can be seen in Figure A1 (Appendix A).
Validation of the simulation program was done in referencing the temperatures in the network given by the simulations to actual production temperatures from 2018 and the temperatures were during winter time. The result of this validation was that when temperatures were below freezing, the actual supply temperatures were between -4 % and +5 % of the value given by the simulation. In the summer time the temperatures were higher than in the simulation by about 10 %. This can be due to heat transfer to the neighboring cities that use a higher DH temperature.
The areas of interest can be seen in Figure 2. The temperature levels are indicated with colors blue, yellow and red. The temperature levels are different in each situation due to the different energy needs at that corresponding outside temperature. The temperature levels are <80 °C (blue), 80-82 °C (yellow) and 82-83 °C (red) when outside temperatures are +14 °C. In outside temperature 0 °C the same values are <91 °C (blue), 91-93 °C (yellow) and 93-94 °C (red). The values for outside temperature of -26 °C can be seen in Figure 3. The other two simulations can be seen in Figure A2 and Figure A3 (Appendix A).
Areas of interest
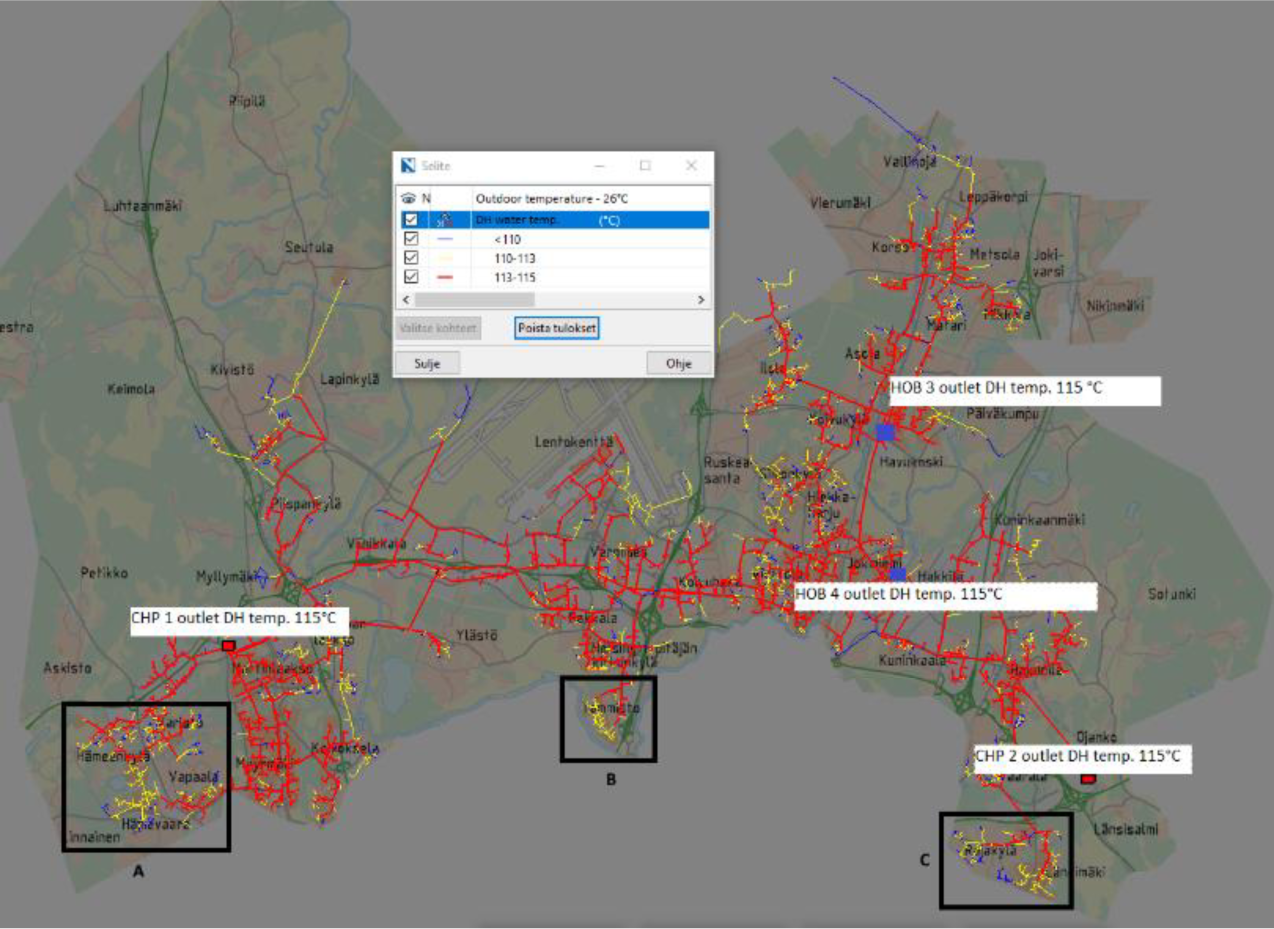
In all three simulations same locations appeared to have maximal decrease in supply temperature which would seem to make these areas favorable places for local production. These areas are marked as Zone A, Zone B and Zone C. These locations are, as expected, on the edges of the network, but surprisingly fairly close to the production units. Especially the southern areas of the network seem to be ideal for local production since these areas have larger parts of the network in lower temperature in the winter when heat is in high demand. The other parts of the network that have higher heat losses are usually due to longer transmission pipe to a neighboring network, a small industrial area or only a few consumers that are further from the main network.
One reason, according to Rämä et al. [27] for the drop in supply temperature could be low heat density in the depicted areas i.e. there is not much consumption in relation to the length of DH pipe. Especially when the heat consumption is very low in the summer, the flow of DH water in the pipes is also low. This leads to bigger heat losses in smaller pipes compared to large ones due to the smaller water volume. However, the increase in heat losses in the summer is a common problem for this type of heating networks in general. These areas offer an interesting possibility for the larger network if supply temperatures can be locally decreased, since this could give the possibility to decrease overall network supply temperatures.
The building stock of the areas presented in Figure 2 was analyzed in order to assess the potential for lowering supply temperatures. The collected building data is presented in Table 1. The zone with the largest building mass was zone A. This area also has the highest share of buildings that are not connected to the DH network. Zone A also has a fairly high share of residential buildings while there are also some retail and logistics buildings. Zone B has a more even division between residential buildings compared to other buildings and it also has the highest share of DH connected buildings. In zone C almost all of the buildings are residential buildings and the percentage of DH connection falls between the two other areas.
Buildings in selected areas
|
Zone A [m2] |
Zone B [m2] |
Zone C [m2] |
---|---|---|---|
All Buildings |
1 075 806 |
295 261 |
448 723 |
Residential |
797 532 |
150 197 |
407 901 |
Office |
18 738 |
3 993 |
0 |
Retail |
110 985 |
90 515 |
4 836 |
Logistics / Industrial |
103 671 |
40 428 |
1 510 |
Public |
30 917 |
3 501 |
30 006 |
Hotels |
5 875 |
0 |
0 |
Others |
8 088 |
1 351 |
4 470 |
Total buildings in District Heating |
727 620 |
246 982 |
326 769 |
Residential |
544 174 |
131 321 |
291 061 |
Office |
18 620 |
3 993 |
0 |
Retail |
101 202 |
90 515 |
4 800 |
Logistics / Industrial |
33 554 |
17 798 |
88 |
Public |
24 063 |
3 169 |
29 575 |
Hotels |
4 500 |
0 |
0 |
Others |
1 507 |
186 |
1 245 |
Share of District Heating |
Zone A [%] |
Zone B [%] |
Zone C [%] |
All Buildings |
68 |
84 |
73 |
Residential |
68 |
87 |
71 |
Office |
99 |
100 |
0 |
Retail |
91 |
100 |
99 |
Logistics / Industrial |
32 |
44 |
6 |
Public |
78 |
91 |
99 |
Hotels |
77 |
0 |
0 |
Others |
19 |
14 |
28 |
The improvement in energy efficiency of buildings has led to lower temperature needs on the secondary side temperatures (building side heating network). The recommended values reflect the buildings maximum values at the rated outside temperature of -26 °C for Southern Finland. For radiator heating for newly built buildings these are +45 °C and +30 °C degrees for supply and return temperatures respectively [28]. The values have drastically dropped from previous rated temperatures. However, the system must always be rated as low as possible without compromising indoor conditions. Rated temperatures for domestic hot water (DHW) heat exchangers are also presented, which are the same for all buildings. In the case heating network a minimum temperature of 65 °C is guaranteed to customers in order to achieve a DHW temperature of 58 °C. These values can be seen in more detail in Table A2 (Appendix A).
Since the construction year of the building affects the rated temperatures for the heating system, the year of connection to the DH network was also studied and can be seen in Figure 3. Zone A has the oldest connections. Zone B has the highest share of newly connected buildings while zones A and C are mainly connected in the 80s and 70s.
Year of DH connection for studied areas
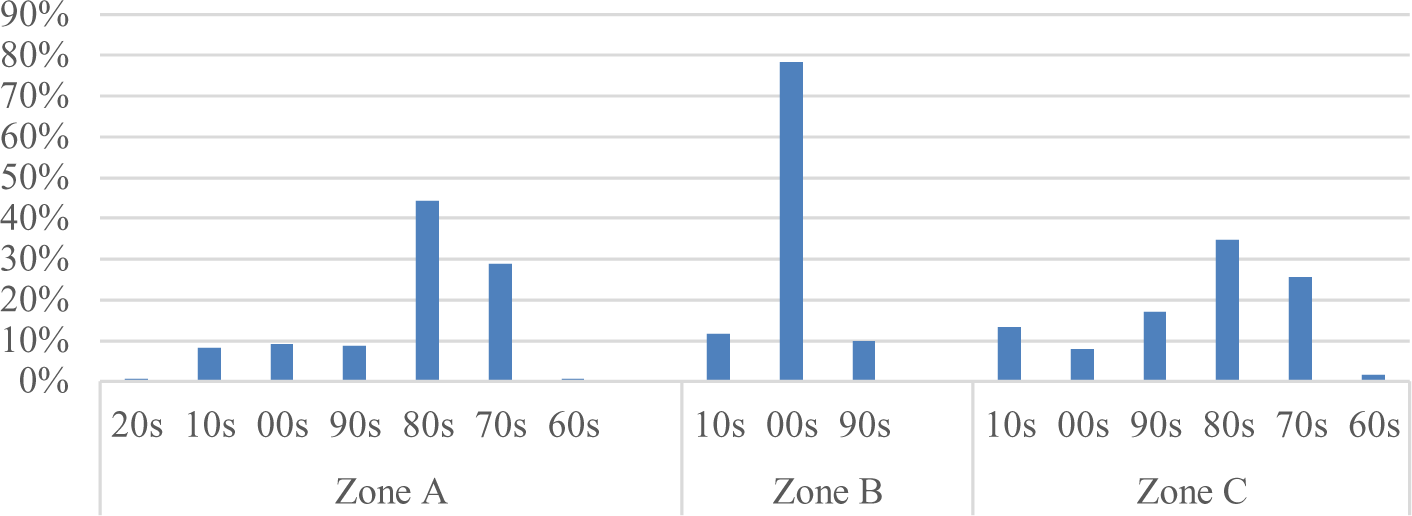
Based on these findings zone B, was taken as a priority due to newest buildings stock. Excess heat production potential is higher retail, logistics and industrial buildings than in residential and office buildings due to the lack of heat emitting processes in office and residential buildings. However, residential and office buildings can utilize heat pumps to recover waste heat from ventilation and waste waters. Commercial buildings can recover waste heat from cooling and other heat intensive processes.
The measured average temperature levels in the network vary between 76 °C and 109 °C on the supply side and between 29 °C and 51 °C on the return side. The absolute minimum temperature level in existing networks in Finland is defined by the heating of DHW which has to be 58 °C to avoid legionella bacteria. Lower levels are possible but require additional heaters on the DHW side. In addition, the flow restrictions of existing networks and customer heat exchangers limit lowering the temperatures at lower outside temperatures. Since the minimum temperature guaranteed in the existing network is 65 °C, this is taken as the lowest supply side temperature.
Reducing the supply temperatures in an existing DH networks has been widely recognized in Finland [29]. However, the progress seems slow even though newer buildings can utilize lower temperatures more efficiently as was also shown in the rated values of heat exchangers. The main issue is that normally networks contain new and old buildings in the same areas. However, areas of new buildings could be separated with a shunt connection or a heat exchanger to create new low temperature networks. Connecting a low temperature network to an existing network has been studied by Volkova et al. [30] on small networks. Dalla Rosa et al. studied separate low temperature networks (supply temperature 60 °C) with a shunt connection and found that a separate network was approximately 4 % more expensive compared to the more traditional network (supply temperature 90 °C), but due to lower operational costs, the end user tariff was almost the same [31]. Mostly due to long distribution distances in conventional DH networks the supply temperature has to be higher than the need of all customers to ensure the heat delivery for everyone in the network. This is one reason why low temperature DH networks need a separate connection to the conventional DH network.
Comparing the actual supply temperatures to the recommended supply temperatures by the local energy company [32], it is estimated that supply temperatures can be decreased especially in the summer. In addition, since the minimum guaranteed temperature is 65 °C, the summer temperatures can be decreased further when temperatures are above 10 °C and heating is used only for DHW. These three different supply curves can be seen in Figure 4. These three scenarios are called Base, Lower and Summer. The return temperature was assumed to decrease the same amount as the supply temperature in Lower and Summer in outside temperatures below 5 °C compared to Base. In Lower at outside temperatures over 5 °C same return temperature was used as in Base. In Summer this was done at outside temperatures over 10 °C. The temperature levels can be seen in Figure 4.
Supply temperature curves
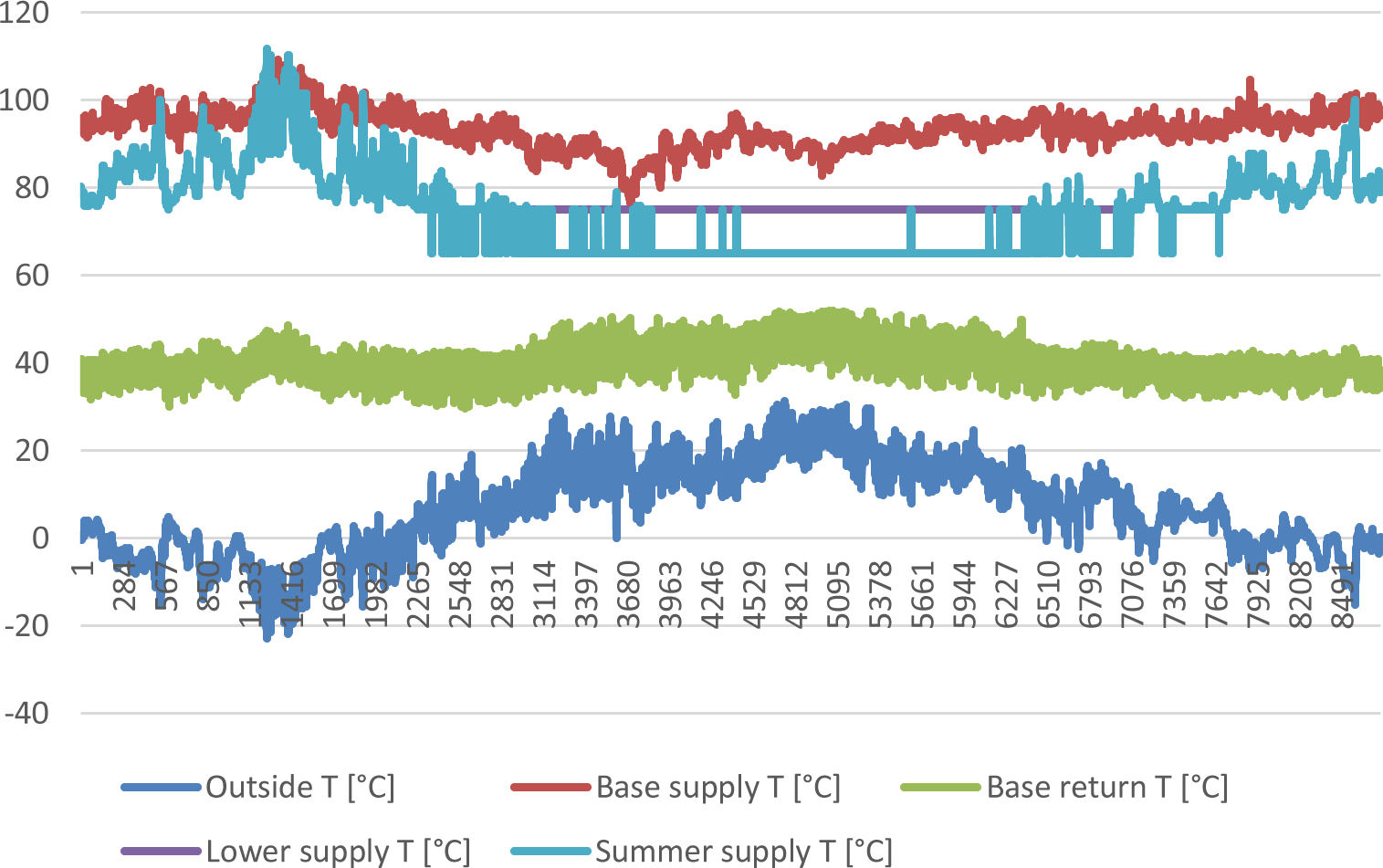
The simulation results were earlier presented in Figure 3. In closer inspection of the marked areas the heat load and losses were simulated with Trimble. The simulation results can be seen in Table 2, where zone A is the western most area in the network. Correspondingly zone B is in the middle and zone C in the east.
Momentary heat load and heat losses of studied zones at simulated temperature
Heat load and loss +14 °C [kW] |
|||
|
Zone A |
Zone B |
Zone C |
Power |
7780 |
1207 |
2130 |
Heat loss |
1620 |
169 |
455 |
Sum |
9400 |
1376 |
2585 |
Heat load and loss 0 °C [kW] |
|||
|
Zone A |
Zone B |
Zone C |
Power |
24651 |
3824 |
6750 |
Heat loss |
3554 |
331 |
1016 |
Sum |
28205 |
4155 |
7766 |
Heat load and loss -26 °C [kW] |
|||
|
Zone A |
Zone B |
Zone C |
Power |
57494 |
9563 |
15743 |
Heat loss |
6891 |
708 |
2153 |
Sum |
64385 |
10271 |
17896 |
The simulation was verified regarding Zone B with actual consumption data from 2018. The actual measurement data corresponds well to the simulation results.
The amount of local heat pump production increased in all real estate types as the supply temperature decreased being at maximum 1214 MWh or 4,6 % of the total annual heat consumption. The results of the heat pump investment optimization are presented for all real estate types in Table 3.
Amount on increased local production
Property type |
Retail |
|||||
Number of heat pumps |
1 |
2 |
||||
Scenario |
Base |
Lower |
Summer |
Base |
Lower |
Summer |
Excess energy produced [MWh] |
91 |
717 |
760 |
67 |
495 |
527 |
Property type |
Residential |
|||||
Number of heat pumps |
1 |
2 |
||||
Scenario |
Base |
Lower |
Summer |
Base |
Lower |
Summer |
Excess energy produced [MWh] |
41 |
313 |
344 |
29 |
242 |
270 |
Property type |
Office |
|||||
Number of heat pumps |
1 |
2 |
||||
Scenario |
Base |
Lower |
Summer |
Base |
Lower |
Summer |
Excess energy produced [MWh] |
2 |
17 |
18 |
2 |
13 |
14 |
Property type |
Logistics / Industry |
|||||
Number of heat pumps |
1 |
2 |
||||
Scenario |
Base |
Lower |
Summer |
Base |
Lower |
Summer |
Excess energy produced [MWh] |
11 |
86 |
92 |
7 |
46 |
50 |
Total produced energy [MWh] |
145 |
1133 |
1214 |
104 |
796 |
862 |
Already at current temperature levels investments into local heat production would be reasonable with a total amount of production being 145 MWh with a single heat pump installation. Production in total increased 782 % compared to Base scenario using temperature levels from Lower scenario. This increase was 839 % compared to Base scenario using temperature levels from Summer scenario. These increases were similar with installations of two heat pumps but the total produced energy was smaller.
In terms of excess heat production and profitability in all real estate types, the production system with one heat pump appears to be better. This is due to the smaller investment. The largest potential of excess heat production was in retail buildings.
The heat pump production was largest in winter between November and February most likely due to higher prices paid for the surplus heat coinciding with low electricity prices. However, this is beneficial for the DH system, since heat demand is largest in the winter. The optimization results are displayed in more detail in Table A4, Table A5, Table A6 and Table A7 (Appendix A).
Hourly heat losses were estimated based on the network information using the actual supply and return temperatures. The change in heat losses were assessed with a network model consisting of actual network data on pipe dimensions and lengths. The values used in the heat loss calculations can be seen in Table 4. The reduction in heat loss was significant decreasing 16.6 % and 22.1 % in the two studied cases compared to the current base scenario.
Heat losses of different scenarios
Scenario |
Heat consumption [MWh] |
Heat losses [MWh] |
Heat losses [%] |
---|---|---|---|
Base |
26678 |
2008 |
7,5 % |
Lower |
26678 |
1674 |
6,3 % |
Summer |
26678 |
1565 |
5,9 % |
The reduction in heat loss and local production also reduces emissions from the overall heating system. Heat loss reduces the need for all production, but it is assumed to decrease DH production. The local heat pump production also replaces DH production but causes emissions because of increased electricity use. However, this will reduce overall emissions because of heat pump efficiency and smaller emission intensity of electricity. Emissions reduced due to smaller losses were 82 498 kgCO2 and 109 421 kgCO2 in scenarios Lower and Summer respectively. The maximum emission reduction from excess heat production was 170 020 kgCO2 in Summer scenario with one heat pump installation. The total emissions of the area in Base case were 7 085 269 kgCO2 so the overall emissions reduction from reduced losses and excess production was 4 %. Emission factor for produced district heat in 2018 in Vantaa was 247 gCO2/kWh [33]. The increased electricity consumption of heat pumps was calculated with an emission factor of 107 gCO2/kWh [34].
Also overall production cost is likely to decrease even though the prosumers are compensated from their production if the pricing of excess heat from prosumers is correctly priced from the energy producer. However, this aspect was not thoroughly investigated in the article.
Understanding the basic fundamentals of DH networks is the first step in developing more efficient and cleaner energy systems. As distributed production that is not based on burning is becoming a necessity, it is imperative to make the most profitable investments first. Two key elements are required for this; the most favourable location and the value of the action. This paper focused on how to evaluate the location for these implementations and the profitability of customer participation in the depicted DH network. The results show that using common network planning tools and open source building data, favourable locations for local production can be found. In addition, when combining real estate investment logic with reduced supply temperatures in a low temperature DH network, profitable local production can be increased by 1214 MWh which is 4,6 % of the total annual heat consumption of the area. These actions also lead to large emissions reductions on a system level.
The goal of this study was to determine drivers for profitable local heat production. One limitation in the calculations of this study is the amount of maximum production. More research should be done on the limit of excess production in a certain area of a DH network. The investment optimization model was a simplification of a complex issue of real estate investment logic into local heat production.
The spatial balancing of the DH network can also lead to savings in energy production on the larger network level. These savings would amount to greater value of local production in certain locations of the heating network compared to other locations.
More research has to be conducted in combining the production and transportation costs of energy in the DH networks. This would aid the assessment of the required magnitude of local production in order to achieve a well-balanced DH network. This involves further research into separating low temperature networks from existing DH networks, as has already been studied and found to be a reasonable investment.
The impact of heat storages, especially seasonal storages, should also be taken into consideration when assessing the value of distributed local production. Storages could also help balance the short term need for heat.
Pricing models of customers and customer centric investments require more research from the DH networks perspective; can customers help in creating new production and balancing the network if the pricing incentive is correct? In order to have holistic pricing for different situations one should consider the value of local production in different temperatures and locations in the DH network, the magnitude, duration and location of DSM measures, seasonal storages (distributed or central) and also the pricing for large scale heat transfer between networks. In addition, prosumers have to make decisions on how and when to produce energy into the network; based on profitability, emissions or balancing the network. This could be done by a multi-objective optimisation on a local level.
Improving the capabilities of the DH network to utilize local production, waste heat and DSM should be studied more closely. The reduction of the network supply temperature would allow for wider temperature range of production possibilities to be utilized. In addition, the impact and value of DSM actions on a local level by different buildings should be studied further as this is an important part of a smart heating system. This is especially important because maximum flows are related to maximum power which is usually reached at the coldest period of the year. This could be mitigated by using DSM to avoid peaks caused by DHW heating.
The restrictions related to reducing supply temperatures has to be understood better. There is a need for data collection from building side heating systems in existing networks in order to understand critical limits and also to optimize the overall energy system. For example fault detection could be used to determine local critical substations. In addition, more research needs to be conducted on the network itself and the effects on it when prosumers change from consuming to producing energy.
Reducing supply temperatures and heat losses in the DH network might also be possible with a heat pump connected to the DHW system. This way the DH network temperature could be lowered and the DHW would still hold at the required temperature level.
I would like to express my gratitude to Vantaa Energy Ltd for the use of consumption and production data for research purposes and also I would like to thank Tomi Virtanen, Pasi Nissinen and Jukka Västinsalo for their comments regarding district heating networks and customer substations.
CHP |
combined heat and power |
COP |
coefficient of performance |
DH |
district heating |
EB |
electric boiler |
EC |
electric connection |
EU |
European Union |
HOB |
heat-only-boiler |
HP |
heat pump (ground source) |
IRR |
internal rate of return |
NPV |
net present value |
OPEX |
operating expenses |
Et |
fixed annual electricity capacity costs |
H |
amount of heat or electricity (kWh) required |
Hdh |
Consumed DH energy |
Heb |
Electricity required for EB |
Hhp |
electricity required for HP |
Hopen |
Energy sold to Open DH |
Ldht |
load demand cost for DH |
Pdht |
price of DH at time t |
Let |
price of electricity |
Lopent |
price received for energy sold to open DH |
r |
discount rate |
t |
lifecycle of the investment (year) |
- European Commission, https://ec.europa.eu/energy/sites/ener/files/documents/1_EN_ACT_part1_v14.pdf
Heat Roadmap Europe: Combining district heating with heat savings to decarbonise the EU energy system ,Energy Policy , Vol. 65 ,pp 475 , 2014, https://doi.org/10.1016/j.enpol.2013.10.035
, - , ECOHEATCOOL Work package 1-The European Heat Market Final Report, Euroheat & Power, Brussels, Belgium, 2006
Heat distribution and the future competitiveness of district heating ,Applied Energy , Vol. 88 (3),pp 568-576 , 2011, https://doi.org/10.1016/j.apenergy.2010.09.020
, - European Commission, A Framework Strategy for a Resilient Energy Union with a Forward-Looking Climate Change Policy, https://eur-lex.europa.eu/resource.html?uri=cellar:1bd46c90-bdd4-11e4-bbe1-01aa75ed71a1.0001.03/DOC_1&format=PDF
- European Commission, Smart cities, Cities using technological solutions to improve the management and efficiency of the urban environment, https://ec.europa.eu/info/eu-regional-and-urban-development/topics/cities-and-urban-development/city-initiatives/smart-cities_en#what-are-smart-cities
4th Generation District Heating (4GDH): Integrating smart thermal grids into future sustainable energy systems ,Energy , Vol. 68 ,pp 1-11 , 2014, https://doi.org/10.1016/j.energy.2014.02.089
, - , Suomalainen kaukolämmitys (Finnish district heating), Veli-Matti Mäkelä J. (Ed)., In: C: Oppimateriaaleja – Study Material 16, Mikkelin ammattikorkeakoulu, 2015
- Energiateollisuus ry - Finnish Energy Industries, Kaukolämpötilastot, https://energia.fi/ajankohtaista_ja_materiaalipankki/tilastot/kaukolampotilastot
Large heat pumps in Swedish district heating systems ,Renewable and Sustainable Energy Reviews , Vol. 79 ,pp 1275-1284 , 2017, https://doi.org/10.1016/j.rser.2017.05.135
, Waste-heat integration in smart thermal networks-A dynamic thermoeconomic approach ,Energy Procedia , Vol. 158 ,pp 6419-6424 , 2019
, - , Open district heating for Espoo city with marginal cost based pricing, IEEE Conference Publications, 2015
Influence of different technologies on dynamic pricing in district heating systems: Comparative case studies ,Energy , Vol. 153 ,pp 136 , 2018
, Prosumers in district heating networks - A Swedish case study ,Applied Energy , Vol. 164 ,pp 492-500 , 2016, https://doi.org/10.1016/j.apenergy.2015.12.020
, Industrial excess heat deliveries to Swedish district heating networks: Drop it like it's hot ,Energy Policy , Vol. 51 ,pp 332 , 2012
, Design and Analysis of District Heating Systems Utilizing Excess Heat in Japan ,Energies , Vol. 12 (7), 2019, https://doi.org/10.3390/en12071202
, Mapping and discussing Industrial Waste Heat (IWH) potentials for different countries ,Renewable and Sustainable Energy Reviews , Vol. 51 ,pp 847-855 , 2015
, Mapping of potential heat sources for heat pumps for district heating in Denmark ,Energy , Vol. 110 ,pp 129-138 , 2016, https://doi.org/10.1016/j.energy.2015.12.127
, Excess heat production of future net zero energy buildings within district heating areas in Denmark ,Energy , Vol. 48 (1),pp 23-31 , 2012, https://doi.org/10.1016/j.energy.2012.04.012
, Regional energy system optimization - Potential for a regional heat market ,Applied Energy , Vol. 86 (4),pp 441-451 , 2009, https://doi.org/10.1016/j.apenergy.2008.09.012
, - , Predictive demand-side management in district heating and cooling connected buildings, M.Sc. Thesis, Aalto University, Espoo, 2016
City Scale Demand Side Management in Three Different-Sized District Heating Systems ,Energies , Vol. 11 (12), 2018, https://doi.org/10.3390/en11123370
, - Ylijäämälämmön taloudellinen hyödyntäminen, https://www.motiva.fi/files/8808/Ylijaamalammon_taloudellinen_hyodynta minen_-_ylijaamalampoenergia-analyysit.pdf.
Smart district heating networks – A simulation study of prosumers’ impact on technical parameters in distribution networks ,Applied Energy , Vol. 129 ,pp 39-48 , 2014, https://doi.org/10.1016/j.apenergy.2014.04.079
, - NEPLAN, https://www.neplan.ch/description/heating-basic-module/
Individual ground source heat pumps: Can district heating compete with real estate owners’ return expectations? ,Sustainable Cities and Society , Vol. 53 ,pp 101982 , 2020
, - , Challenges on low heat density district heating network design, Proceedings of the 12th International Symposium on District Heating and Cooling, 2010
- Energiateollisuus ry - Finnish Energy Industries, District heating of buildings Regulations and guidelines Publication K1/2013, https://energia.fi/files/1555/DH_of_buildings_PublicationK1_EN.pdf
Towards next generation district heating in Finland ,Renewable and Sustainable Energy Reviews , Vol. 65 ,pp 915-924 , 2016
, Small low-temperature district heating network development prospects ,Energy , Vol. 178 ,pp 714 , 2019, https://doi.org/10.1016/j.energy.2019.04.083
, District heating (DH) network design and operation toward a system-wide methodology for optimizing renewable energy solutions (SMORES) in Canada: A case study ,Energy , Vol. 45 (1),pp 960-974 , 2012, https://doi.org/10.1016/j.energy.2012.06.062
, - Prices and terms on waste heat (In Finnish: Hukkalämmön ehdot ja hinnat), https://www.vantaanenergia.fi/lampo/myy-hukkalampo-eteenpain-avoimeen-kaukolampoverkkoomme/hukkalammon-ehdot-ja-hinnat/
- Emission factor for produced district heat in Vantaa. Corporate responsibility report 2018, https://www.vantaanenergia.fi/ykv/ykv-2018/sahkon-lammon-tuotanto/
- Energy year 2019 (In Finnish Energiavuosi 2019), https://energia.fi/julkaisut/materiaalipankki/energiavuosi_2019_sahko.html#material-view)
- , Datscha, Data for property market yields, 2018
- Oilon Oy, https://oilon.com/en-us
- One1, https://s3-eu-west-1.amazonaws.com/frantic/vantaanenergia/uploads/20170328090837/Kilpailukykyvertailuone1.pdf
- Termax, https://termocal.fi/
- Vantaa Energy Electricity Grid, Prices, https://www.vantaanenergiasahkoverkot.fi/en/
- Vantaa Energy, District heating prices, https://www.vantaanenergia.fi/lampo/hinnastot-ja-ehdot/
- Statistics Finland, http://www.stat.fi/til/ehi/index.html
- Verohallinto, https://www.vero.fi/en/businesses-and-corporations/about-corporate-taxes/excise_taxes/valmisteverolajit/sahko_ja_eraat_polttoaineet/s%C3%A4hk%C3%B6n-ja-er%C3%A4iden-polttoaineiden-verotaulukot/
- Nordpool, 2017, http://www. nordpoolspot.com/Market-data1/
- Vantaa City Surveying Department, https://hri.fi/fi/
- Vantaa Energy, 2018, https://www.vantaanenergia.fi/en/